Maximizing Credit Card Conversion Through First-Party Data
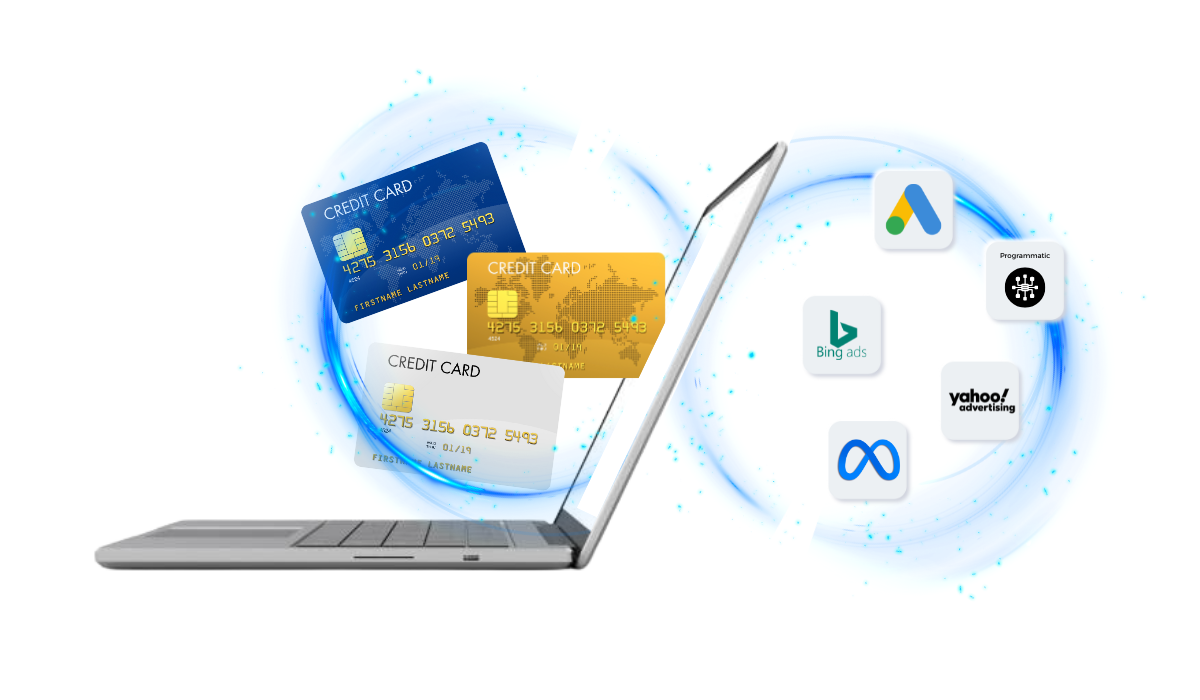
The Business Challenge
As Malaysia gradually returned to post-pandemic life, consumer spending habits saw a positive return to pre-pandemic levels, indicated by a significant increase in credit card usage and the 41.51% increase in credit card-related keywords in 2022. In the second half of the year, a leading local bank recorded a 40% surge in search demand, outperforming the two largest banks in the country.
However, the consumer landscape had already changed significantly.
Although consumer spending was on a rebound, it manifested in new and different ways. To begin with, digital adoption had increased, and with it came increased demand on digital channels–like search–for core banking products. This growth also led to aggressive and dynamic competition; the Finance and Banking industry (FSI) sector was one of only two industries (alongside retail) to see increased ad spends in the previous year, with spends rising by 28% between Q1 2021 and Q2 2022.
Secondly, Malaysian search habits had also evolved. Google Finance observed a 700% increase in searches for “how to save money fast on low income”, and a 321% increase in “savings bank account”, but also noted large increases in searches for travel and travel-related products. This painted a complex picture of the Malaysian consumer landscape, where grim economic concerns existed side-by-side with rebound demand for opportunities lost to the pandemic.
In mid-2022, the bank found itself 60% below their lead target in mid-2022, with major platforms showing diminishing returns and creative fatigue.
Solution
To reverse the trend, the bank needed to increase the consideration of the targeted audience by appearing in every customer journey touchpoints, identifying and targeting high-intent audiences at a low CPL, and ultimately, generating as much as possible quality website leads from their credit card landing page.
We started by defining our audience. Our analysis uncovered three pivotal moments that reinforce customers’ drive to own a credit card – shopping, travel, and essential purchases. We centered our messaging and targeting among the audience that fall under these interests and have interest in banking products.
With a refined target audience, the following strategies were laid out:
- Increase product awareness and improve acquisition by implementing an omnichannel campaign
- Supercharge acquisition strategy and enable cross-channel marketing ads by collecting first-party data and identifying customer-match audience data
Implementation
To achieve our business objectives, we defined our platform based on two goals:
- Traffic Driver - gather first-party data by capturing low-CPC website visitors from GDN, Programmatic, and Yahoo Native channels.
- Lead Driver - merge prospecting and remarketing audiences who convert from Google and Bing Search, Discovery, Performance Max, and Meta.
We simultaneously ran Discovery, GDN, and Performance Max campaigns to boost the bank's relevance at every digital touchpoint and supply data to the machine learning algorithms. This helped find high-quality audiences and optimize smart bidding, maximising budget utilisation. Non-last-click attribution model was used to recognise all ad interactions that led to conversion.
We used our customer list to define remarketing audiences from branding campaigns and similar audiences from other card products, allowing us to target and cross-sell credit card products to existing customers who haven’t owned any yet.
To increase spending without jeopardizing efficiency, we implemented a ‘Portfolio Bidding Strategy’ to group search campaign bids and achieve the desired CPA results.
And to ensure our creative are relevant to the target audience, we integrated the shopping, travel, and essential purchases into our advertising messaging making it more customer-centric. From just showcasing the card's appearance and common benefits like "No Annual Fee," we amplified its unique advantages.
We tested an ad highlighting all benefits against variants focusing on individual benefits. Using the machine’s learning, we identified and reallocated the budget to winning variants. We refreshed our creative content four times on Facebook and three times on Google, resulting in a 46.72% and a 31% reduction in CPL respectively.
Growth Results
.gif?width=1200&height=1200&name=Case%20Study%20National%20Bank%20Results%201%20(1).gif)
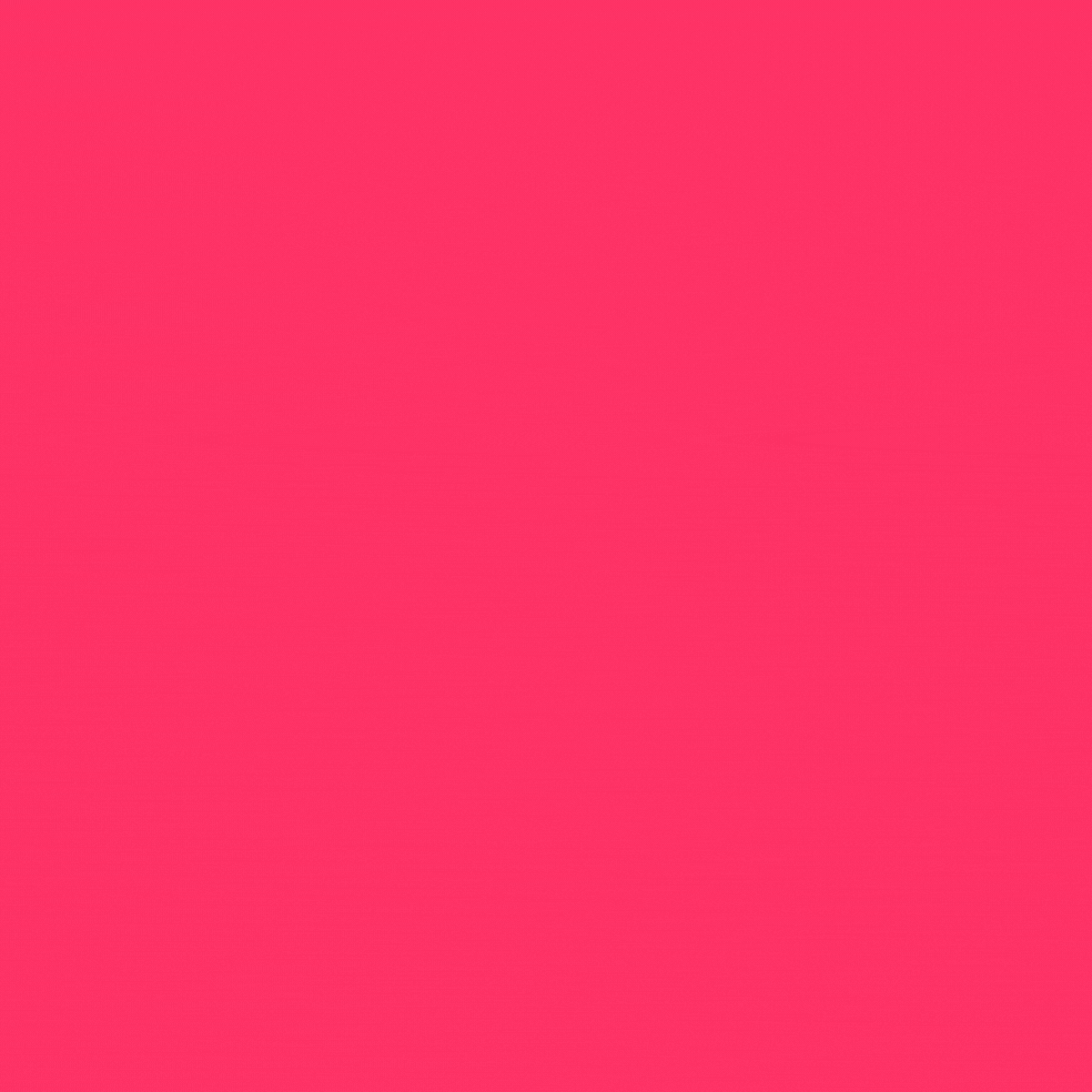
.gif?width=1200&height=1200&name=Case%20Study%20National%20Bank%20Results%201%20(2).gif)
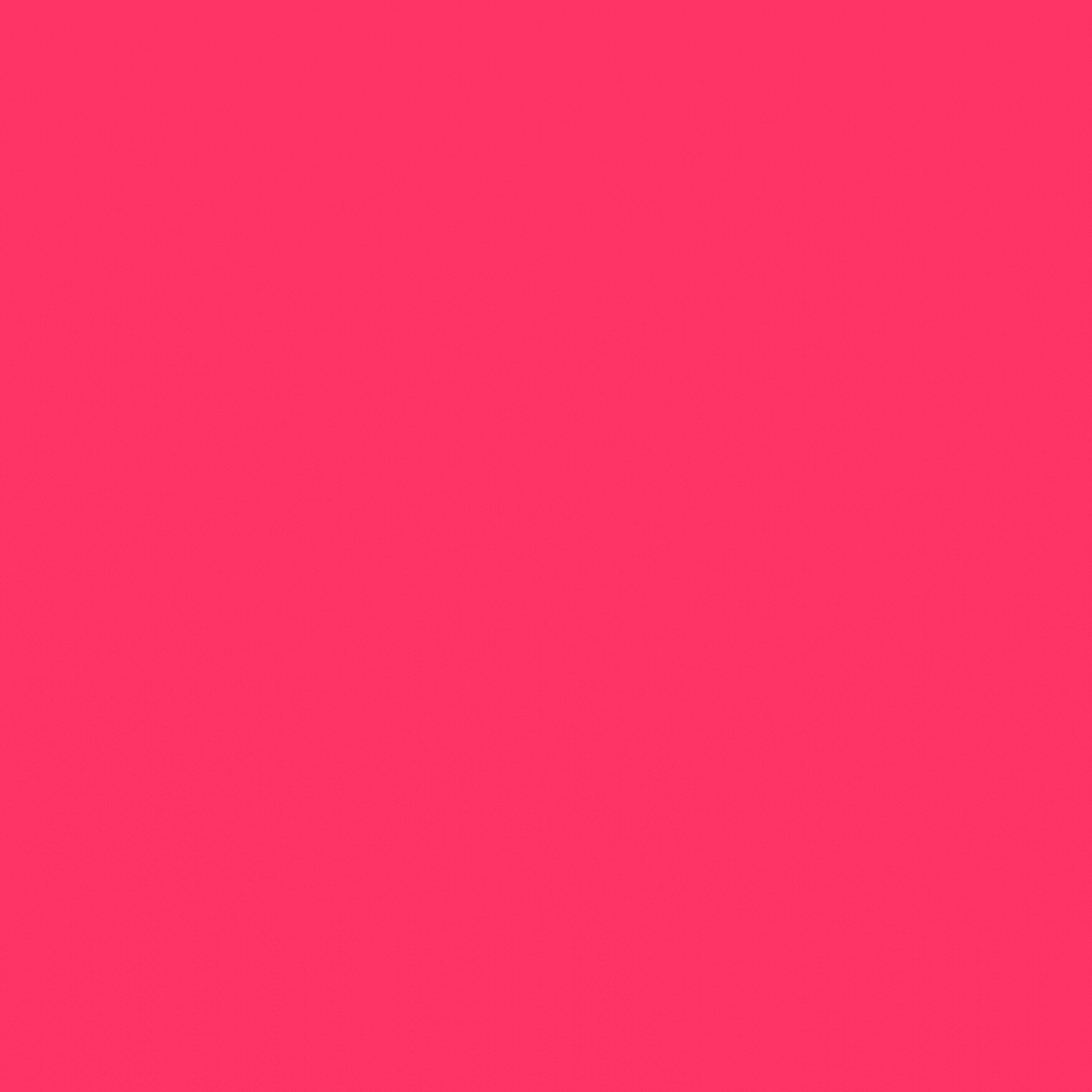
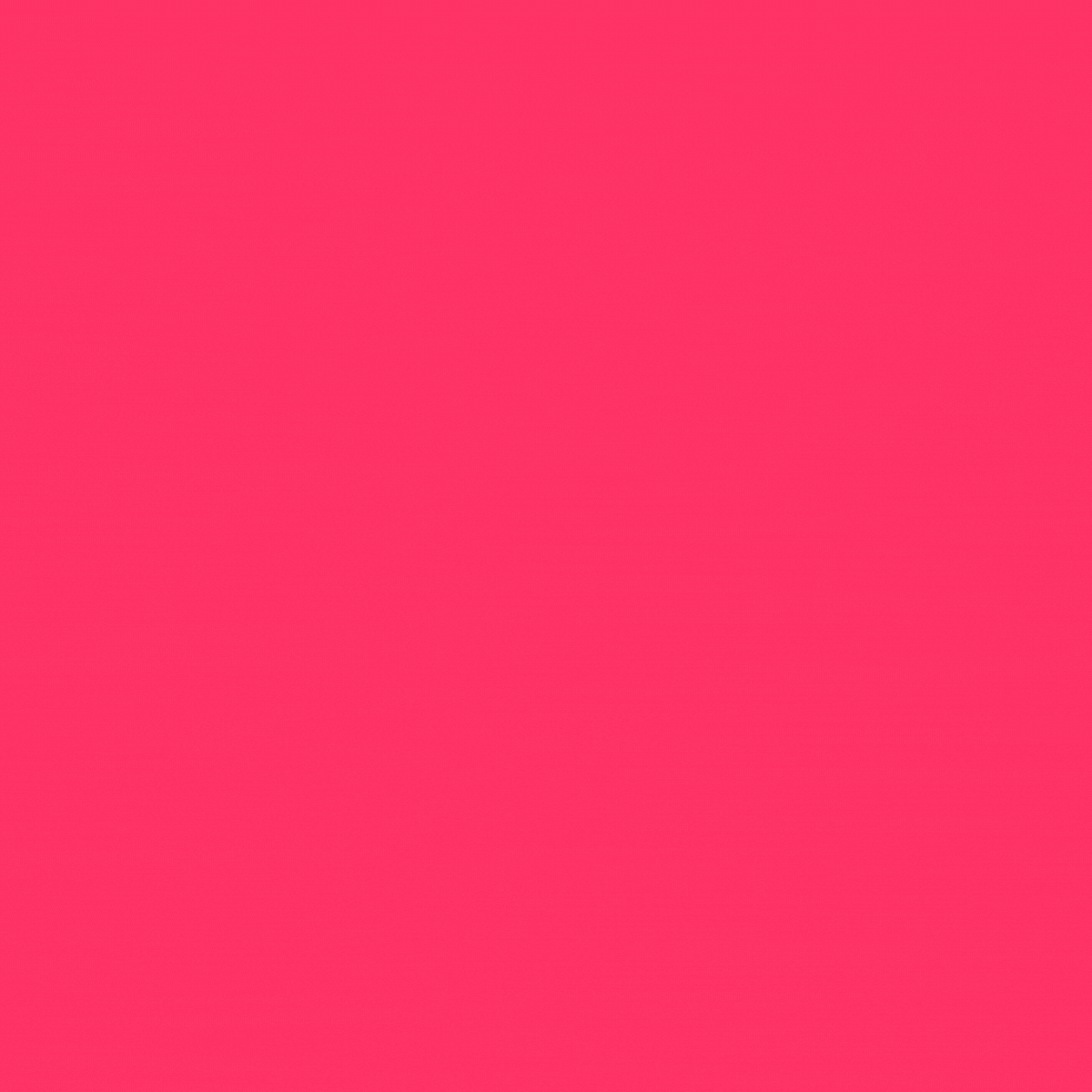
.gif?width=1200&height=1200&name=Case%20Study%20National%20Bank%20Results%205%20(1).gif)